
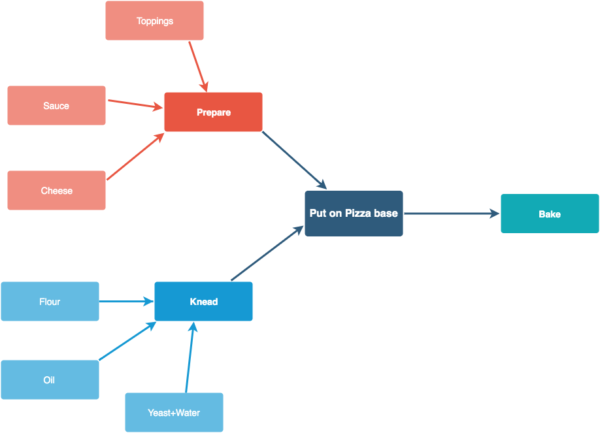
Its well-defined architecture allows for high availability and strong security controls.Airflow’s proponents consider it to be distributed, scalable, flexible, and well-suited to handle the orchestration of complex business logic.Highly extensible and plays well with a variety of data processing tools and services.Advantages of Airflow’s dynamic pipeline generation Airflow is built using hooks to abstract information Airflow operators generate tasks that become nodes in a DAG, and executors (usually Celery) run jobs remotely and handle message queuing. Hooks and executors in the Airflow environment: Hooks are pieces of code that are invoked by operators to interact with databases, servers, and external services. DAGs can be created from configuration files or other metadata.

Tasks are then grouped together to form DAGs. Operators can be grouped together to form upstream tasks. DAGs are composed of operators, which are nodes in the graph that represent an individual task. How Airflow Works – Build and Monitor WorkflowsĭAGs: Airflow enables you to manage your data pipelines by authoring and monitoring workflows as Directed Acyclic Graphs (DAGs) of tasks, which instantiates pipelines dynamically. The goal of the project was to enable greater productivity and better workflows for data engineers. Airflow is written in Python and uses the Django web framework.
Apache airflow python tutorial software#
The open-source distribution is available through the Apache Software Foundation.Īirflow was originally created by Airbnb and was open sourced in June 2015. Airflow is an open-source workflow management system designed to programmatically author, schedule, and monitor data pipelines and workflows.
